Pythonでの使用例
画像を取得して表示する
次のPythonスクリプトは、画像を取得して表示するというAPIの中で最も簡易的な使用例です。ユーザーが引数を入力しない場合は、各メソッドのデフォルト値が使用されます。ssl エラーが発生した場合は、ssl_verifyを False
に設定してください。
# モジュールをロード
from jaxa.earth import je
# 画像を取得
data = je.ImageCollection(ssl_verify=True)\
.filter_date()\
.filter_resolution()\
.filter_bounds()\
.select()\
.get_images()
# 画像を処理して表示
img = je.ImageProcess(data)\
.show_images()
以下の画像が表示されます。
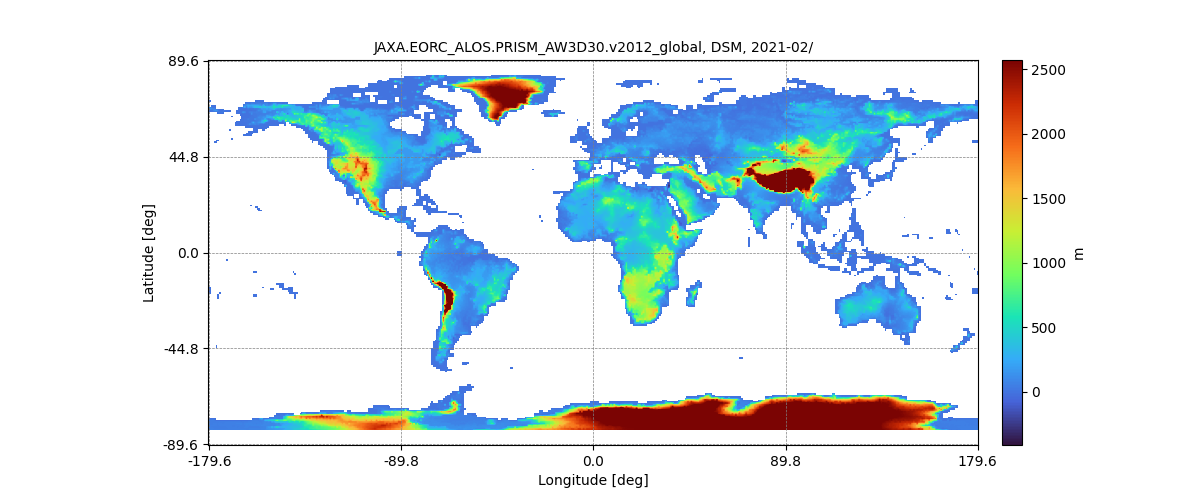
生のNumPy配列を取得して表示したい場合は、次のスクリプトを実行してください。
import matplotlib.pyplot as plt
plt.imshow(img.raster.img[0])
plt.show()
以下の画像が表示されます。
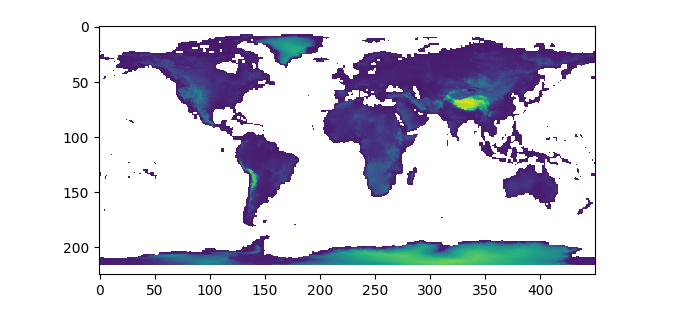
コレクションIDとバンドを検索
全てのコレクションIDとバンドを知りたい場合はこちらのページをご確認ください。
https://data.earth.jaxa.jp/en/datasets/
ImageCollectionList
クラスの filter_name
メソッドにキーワードのリストを入れることで、コレクションのformal IDとバンドを簡単に検索し、特定することができます。
関心領域ごとに画像を取得して表示する
次に、kw(コレクションIDからコレクションを検出するためのキーワード)、dlim(期間の範囲)、ppu(pixels per unit(1度))などの各パラメータを設定してください。
bboxによる関心領域の設定
bbox(バウンディングボックス)を設定すると、指定した領域が表示されます。
# モジュールをロード
from jaxa.earth import je
# クエリパラメータを設定
kw = ["Aqua","LST","half-monthly"]
dlim = ["2021-01-01T00:00:00","2021-01-01T00:00:00"]
ppu = 5
bbox = [110, 20, 160, 50]
# コレクションとバンドの情報を取得
collections,bands = je.ImageCollectionList(ssl_verify=True)\
.filter_name(keywords=kw)
# 画像を取得
data = je.ImageCollection(collection=collections[0],ssl_verify=True)\
.filter_date(dlim=dlim)\
.filter_resolution(ppu=ppu)\
.filter_bounds(bbox=bbox)\
.select(band=bands[0][0])\
.get_images()
# 画像を処理して表示
img = je.ImageProcess(data)\
.show_images()
以下の画像が表示されます。
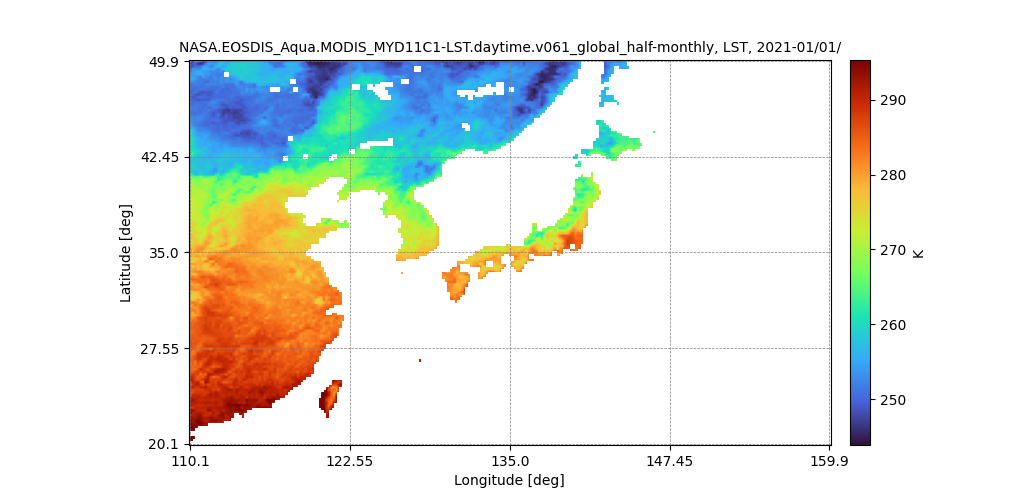
geojsonによる関心領域の設定
お使いのコンピューターからgeojsonデータを読み込んで設定すると、要求されたエリアが表示されます。ユーザーはウェブサービス(geojson.io)でgeojsonデータを作成するか、QGISでシェイプファイルなどの他のベクターフォーマットから変換することができます。
# モジュールをロード
from jaxa.earth import je
# クエリパラメータを設定
kw = ["Aqua","LST","half-monthly"]
dlim = ["2021-01-01T00:00:00","2021-01-01T00:00:00"]
ppu = 20
# コレクションとバンドの情報を取得
collections,bands = je.ImageCollectionList(ssl_verify=True)\
.filter_name(keywords=kw)
# FeatureCollectionデータを取得
geoj_path = "test.geojson"
geoj = je.FeatureCollection().read(geoj_path).select()
# 画像を取得
data = je.ImageCollection(collection=collections[0],ssl_verify=True)\
.filter_date(dlim=dlim)\
.filter_resolution(ppu=ppu)\
.filter_bounds(geoj=geoj[0])\
.select(band=bands[0][0])\
.get_images()
# 画像を処理して表示
img = je.ImageProcess(data)\
.show_images()
以下の画像が表示されます。
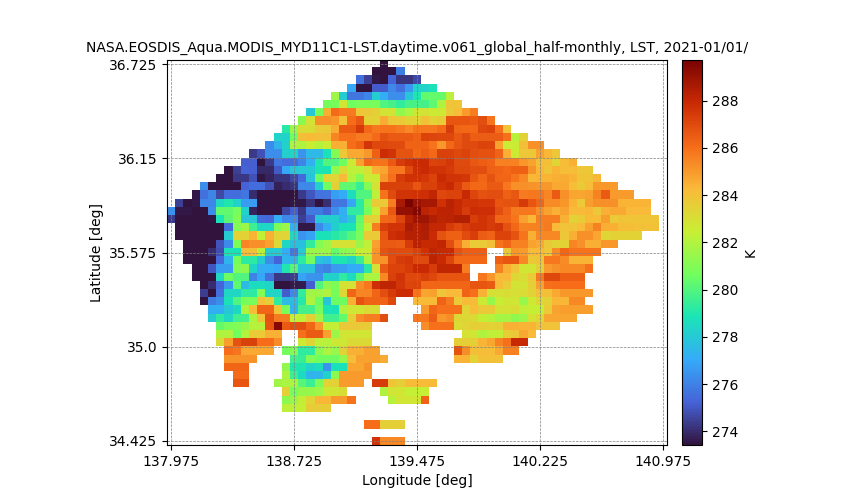
マスクされた画像を取得して表示する
次に、 ImageProcess
クラスオブジェクトの mask_images
メソッドを使用してデータをマスクします。マスクするデータ(マスク用データとマスクされるデータ)の2種類を取得する必要があります。
範囲データのピクセルを抽出する
次に、データとしてGSMaP (Monthly Rain rate)、マスキングにはAW3D DSM (Elevation) を使用しています。GSMaPデータは、AW3Dのrange 0.01から1000までで抽出されます。
# モジュールをロード
from jaxa.earth import je
# クエリパラメータを設定
kw_d = ["GSMaP","monthly"]
kw_m = ["AW3D"]
dlim = ["2021-02-01T00:00:00","2021-02-01T00:00:00"]
ppu = 10
bbox = [110, 20, 160, 50]
mq = "range"
val = [0.1,1000]
# コレクションとバンドの情報を取得
collections_d,bands_d = je.ImageCollectionList(ssl_verify=True)\
.filter_name(keywords=kw_d)
# データ用の画像を取得する
data_d = je.ImageCollection(collection=collections_d[0],ssl_verify=True)\
.filter_date(dlim=dlim)\
.filter_resolution(ppu=ppu)\
.filter_bounds(bbox=bbox)\
.select(band=bands_d[0][0])\
.get_images()
# マスク用のコレクション、バンドの情報を取得
collections_m,bands_m = je.ImageCollectionList(ssl_verify=True)\
.filter_name(keywords=kw_m)
# マスク用の画像の取得
data_m = je.ImageCollection(collection=collections_m[0],ssl_verify=True)\
.filter_date(dlim=dlim)\
.filter_resolution(ppu=ppu)\
.filter_bounds(bbox=bbox)\
.select(band=bands_m[0][0])\
.get_images()
# 画像を処理して表示
img = je.ImageProcess(data_d)\
.mask_images(data_m,method_query=mq, values=val)\
.show_images()
以下の画像が表示されます。
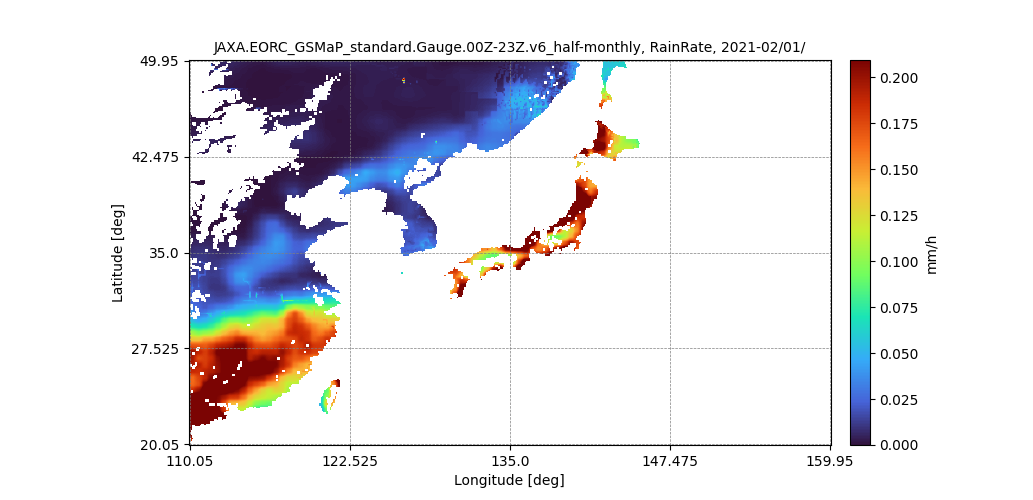
等しいデータのピクセル値を抽出する
次に、データとしてAW3D DSM (Elevation)、マスキングにはLCCS (Land cover) を使用しています。AW3Dのデータは、LCCSの190 (Urban areas)の値によって抽出されます。
# モジュールをロード
from jaxa.earth import je
# クエリパラメータを設定
kw_d = ["AW3D"]
kw_m = ["LCCS"]
dlim = ["2019-01-01T00:00:00","2021-02-01T00:00:00"]
ppu = 360
bbox = [139.5, 35, 140.5, 36]
mq = "values_equal"
val = [190]
# コレクションとバンドの情報を取得
collections_d,bands_d = je.ImageCollectionList(ssl_verify=True)\
.filter_name(keywords=kw_d)
# データ用の画像を取得する
data_d = je.ImageCollection(collection=collections_d[0],ssl_verify=True)\
.filter_date(dlim=dlim)\
.filter_resolution(ppu=ppu)\
.filter_bounds(bbox=bbox)\
.select(band=bands_d[0][0])\
.get_images()
# マスク用のコレクション、バンドの情報を取得
collections_m,bands_m = je.ImageCollectionList(ssl_verify=True)\
.filter_name(keywords=kw_m)
# マスク用の画像の取得
data_m = je.ImageCollection(collection=collections_m[0],ssl_verify=True)\
.filter_date(dlim=dlim)\
.filter_resolution(ppu=ppu)\
.filter_bounds(bbox=bbox)\
.select(band=bands_m[0][0])\
.get_images()
# 画像を処理して表示
img = je.ImageProcess(data_d)\
.mask_images(data_m,method_query=mq, values=val)\
.show_images()
以下の画像が表示されます。
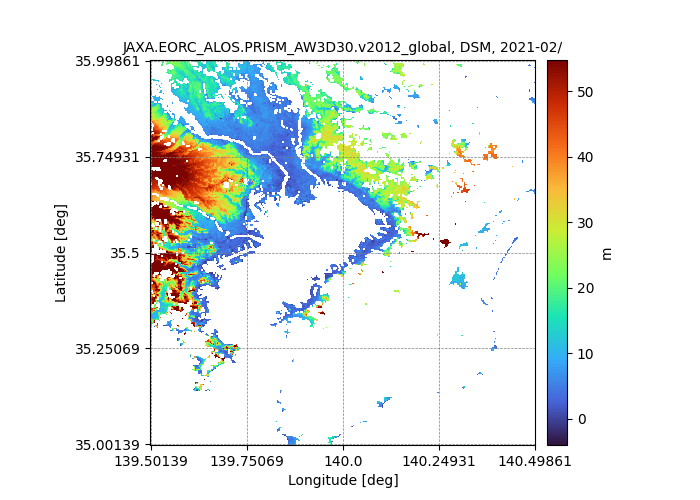
等しいビットのデータピクセルを抽出する
次に、AW3D DSM(Elevation)とMSK(Mask)がそれぞれがデータとマスクとして使用しています。AW3Dのデータバンドには、DSM(Elevation)とMSK(Mask)の2つのバンドがあります。DSMのデータは、MSKのビットが全てゼロ(有効画素数)の場合抽出されます。
# モジュールをロード
from jaxa.earth import je
# クエリパラメータを設定
kw = ["AW3D"]
dlim = ["2019-01-01T00:00:00","2021-02-01T00:00:00"]
ppu = 360
bbox = [139.5, 35, 140.5, 36]
mq = "bits_equal"
val = [0,0,0,0,0,0,0,0]
# コレクションとバンドの情報を取得
collections,bands = je.ImageCollectionList(ssl_verify=True)\
.filter_name(keywords=kw)
# データ用の画像を取得する
data_d = je.ImageCollection(collection=collections[0],ssl_verify=True)\
.filter_date(dlim=dlim)\
.filter_resolution(ppu=ppu)\
.filter_bounds(bbox=bbox)\
.select(band="DSM")\
.get_images()
# マスク用の画像の取得
data_m = je.ImageCollection(collection=collections[0],ssl_verify=True)\
.filter_date(dlim=dlim)\
.filter_resolution(ppu=ppu)\
.filter_bounds(bbox=bbox)\
.select(band="MSK")\
.get_images()
# 画像を処理して表示
img = je.ImageProcess(data_d)\
.mask_images(data_m,method_query=mq, values=val)\
.show_images()
以下の画像が表示されます。
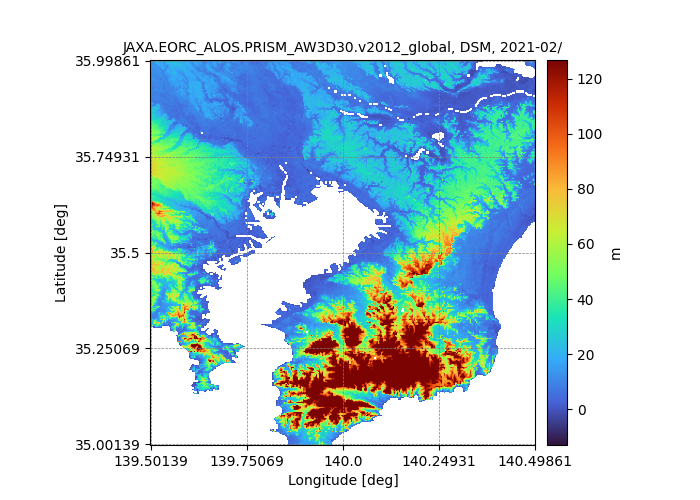
差分画像を取得して表示する
次に、 ImageProcess
クラスの diff_images
メソッドを使い、差分データを取得しています。2種類のデータ(比較対象となるデータと基準データ)を取得する必要があります。この例では対象データとしてNDVI (Aqua, monthly)、基準データとしてNDVI (Aqua, monthly-normal)を使用しています。
# モジュールをロード
from jaxa.earth import je
# クエリパラメータを設定
kw_d = ["ndvi","_monthly"]
kw_r = ["ndvi","_monthly","normal"]
dlim = ["2021-08-01T00:00:00","2021-08-01T00:00:00"]
# データ用のコレクション、バンドの情報を取得
collections_d,bands_d = je.ImageCollectionList(ssl_verify=True)\
.filter_name(keywords=kw_d)
# データ用の画像を取得する
data_d = je.ImageCollection(collection=collections_d[0],ssl_verify=True)\
.filter_date(dlim=dlim)\
.filter_resolution()\
.filter_bounds()\
.select(band=bands_d[0][0])\
.get_images()
# Collectionとバンドの情報を取得
collections_r,bands_r = je.ImageCollectionList(ssl_verify=True)\
.filter_name(keywords=kw_r)
# 基準画像を入手する
data_r = je.ImageCollection(collection=collections_r[0],ssl_verify=True)\
.filter_date(dlim=dlim)\
.filter_resolution()\
.filter_bounds()\
.select(band=bands_r[0][0])\
.get_images()
# 画像を処理して表示
img = je.ImageProcess(data_d)\
.diff_images(data_r)\
.show_images()
以下の画像が表示されます。
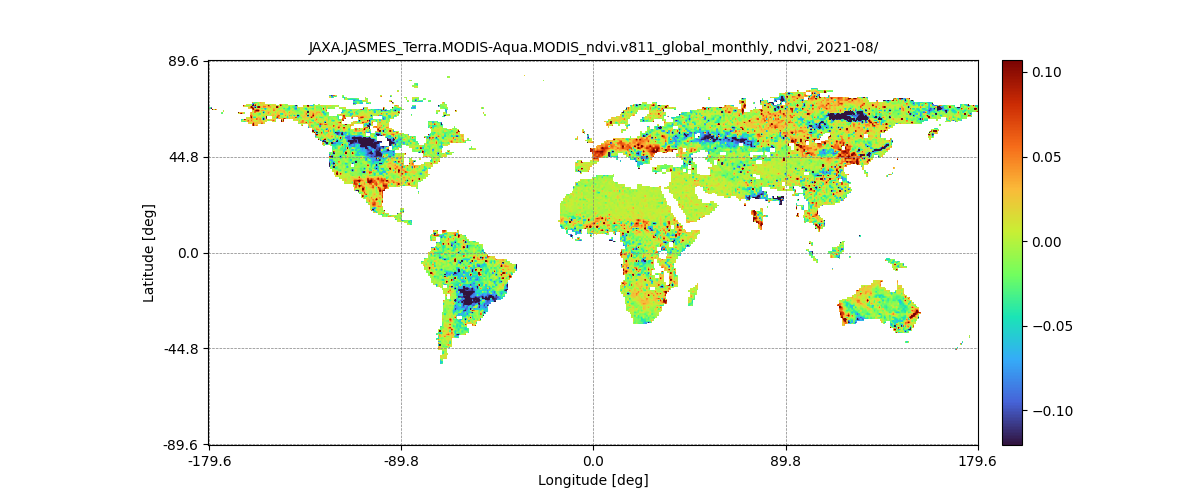
合成画像を取得して表示する
次に、 ImageProcess
クラスの calc_temporal_stats
メソッドを使用して、合成画像を取得しています。この例では、SMC (Soil Moisture Content, daily)が合成の対象として使用されています。
# モジュールをロード
from jaxa.earth import je
# クエリパラメータを設定
kw_d = ["SMC","_daily"]
dlim = ["2021-08-01T00:00:00","2021-08-10T00:00:00"]
# データ用のコレクション、バンドの情報を取得
collections,bands = je.ImageCollectionList(ssl_verify=True)\
.filter_name(keywords=kw_d)
# データ用の画像を取得する
data = je.ImageCollection(collection=collections[0],ssl_verify=True)\
.filter_date(dlim=dlim)\
.filter_resolution()\
.filter_bounds()\
.select(band=bands[0][0])\
.get_images()
# 画像を処理して表示
img = je.ImageProcess(data)\
.calc_temporal_stats("mean")\
.show_images()
以下の画像が表示されます。
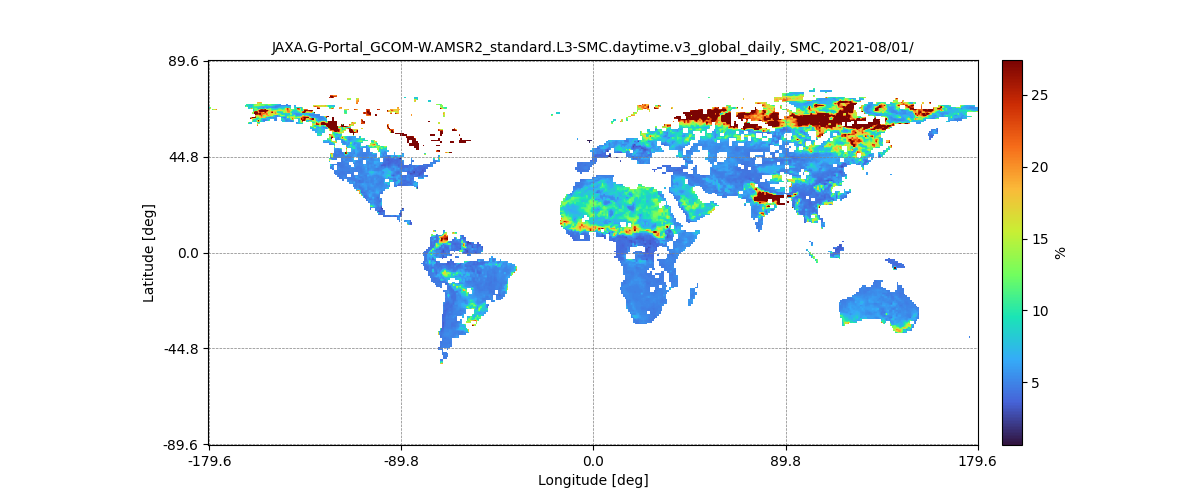
時系列データの計算と表示
最後に、 ImageProcess
クラスのオブジェクトの calc_spatial_stats
メソッドと show_spatial_stats
メソッドを使用して、空間統計を計算し、表示します。この例では、swr (Short Wave Radiation, half-monthly-normal)を使用しています。
# モジュールをロード
from jaxa.earth import je
# クエリパラメータを設定
kw_d = ["swr","half-monthly-normal"]
dlim = ["2021-01-01T00:00:00","2021-12-31T00:00:00"]
bbox = [120,20,150,50]
ppu = 10
# データ用のコレクション、バンドの情報を取得
collections,bands = je.ImageCollectionList(ssl_verify=True)\
.filter_name(keywords=kw_d)
# データ用の画像を取得する
data = je.ImageCollection(collection=collections[0],ssl_verify=True)\
.filter_date(dlim=dlim)\
.filter_resolution(ppu=ppu)\
.filter_bounds(bbox=bbox)\
.select(band=bands[0][0])\
.get_images()
# 画像を処理して表示
img = je.ImageProcess(data)\
.calc_spatial_stats()\
.show_spatial_stats()
以下の時系列グラフが表示されます。
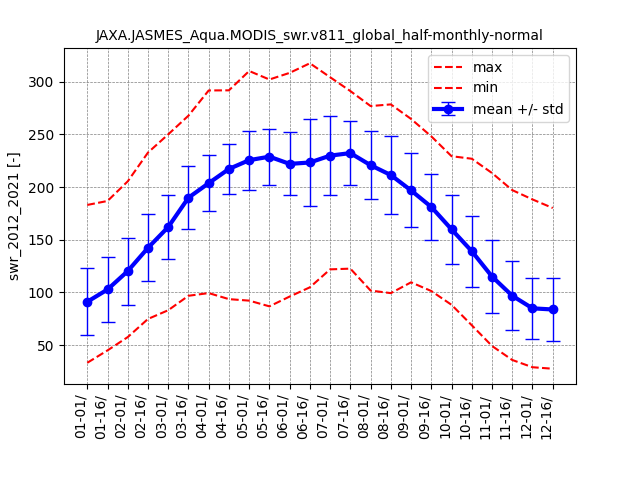